TheNZThrower
Active Member
According to a Cato Institute article by Randal O'Toole, the effects of induced demand are exaggerated and insignificant.
He beings by claiming that a Wired magazine article he is responding to misrepresents a 2009 study by economists Turner and Duranton - published in the peer-reviewed journal American Economic Review - they cited.
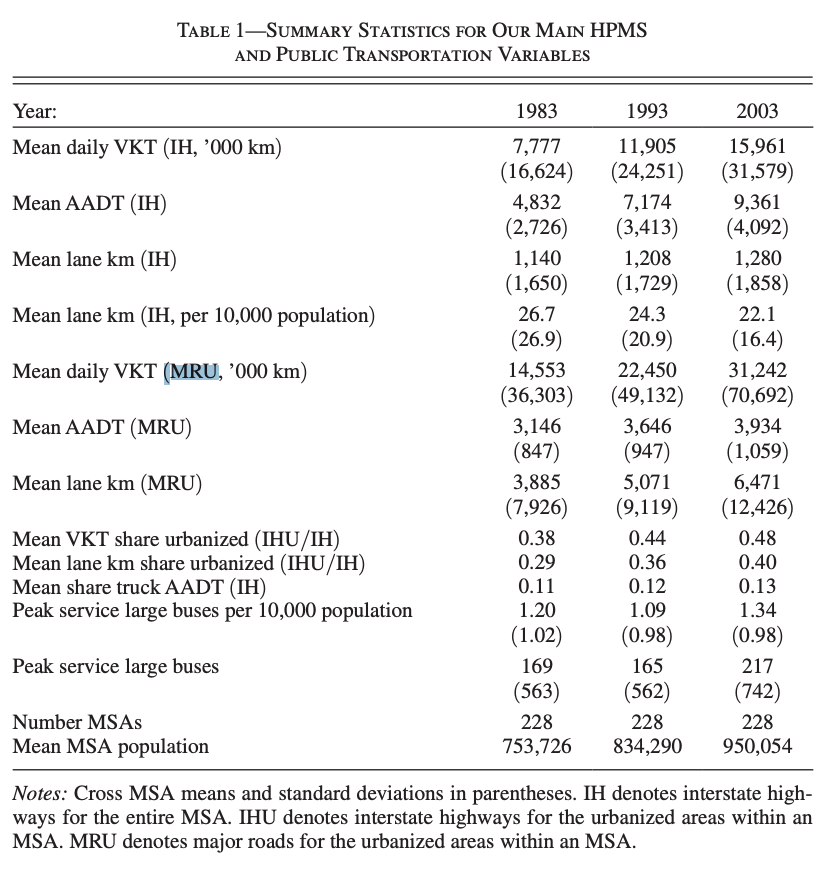
The increase in VKT and lane km on the Interstate Highway (AKA: IH) is as follows:
If we adjust those figures respectively for the percentage of VKT and lane km that's urbanised, we get 2,955,260 (6,317,120) km for 1983, 5,238,200 km (10,670,440) for 1993, and 7,661,000 (15,157,920) km for 2003; and 330.6 (478.5) km for 1983, 434.8 (622.4) km for 1993, and 512 (743.2) km for 2003. Now we can calculate the change in VKT in urbanised areas:
Now we can calculate the lane km growth percentage vs VKT growth percentage:
So Randal's initial statement that:
In fact, Randal did leave out the following data from Giles & Duranton:
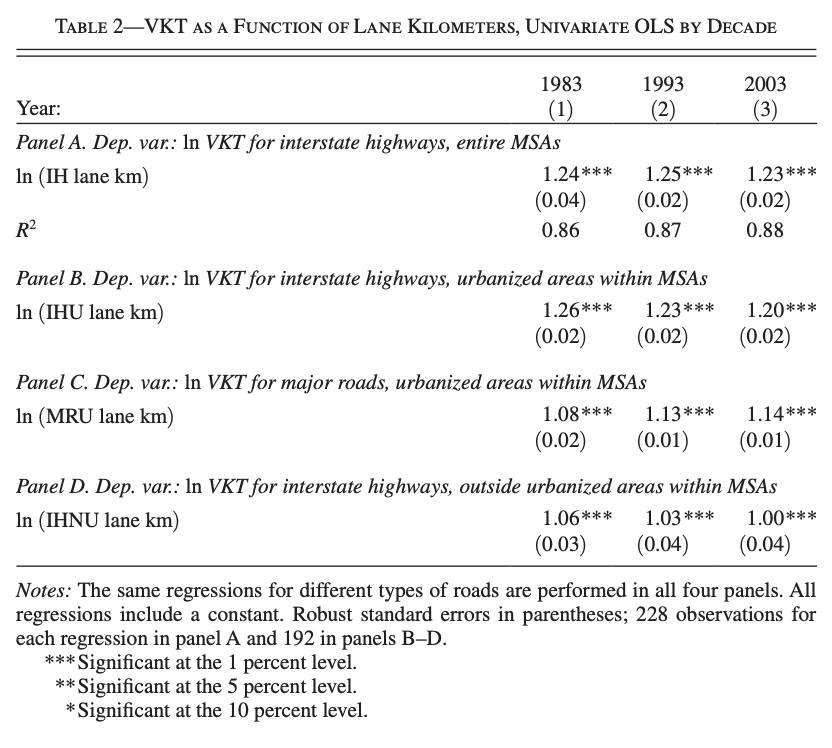
So evidently Randal used a childishly simplistic comparison between the % change in VKT & lane km to determine the elasticity between the two variables, when an Ordinary Least Squares (OLS) regression is more appropriate in this context, and gives much different results.
Now I don't know what OLS is, and the math in Giles & Duranton's paper is way above my head, but I think that two economists trained in their field probably know quite a bit more than is required to be capable of such a brazen contradiction that Randal alleges. Ergo, if you spot a contradiction - real or not - in a peer reviewed paper written by experts in the relevant field, chances are they would have done the same, as it is highly unusual for them to miss such a simple contradiction.
He beings by claiming that a Wired magazine article he is responding to misrepresents a 2009 study by economists Turner and Duranton - published in the peer-reviewed journal American Economic Review - they cited.
However, according to the abstract, there is a 1:1 relationship:“Building bigger roads actually makes traffic worse,” asserts Wired magazine. “The reason you’re stuck in traffic isn’t all these jerks around you who don’t know how to drive,” says writer Adam Mann...
In support of the induced‐demand claim, Mann cites research by economists Matthew Turner of the University of Toronto and Gilles Duranton of the University of Pennsylvania. “We found that there’s this perfect one‐to‐one relationship,” Mann quotes Turner as saying.
However, this is simply not true. Nor is it what Duranton & Turner’s paper actually said. The paper compared daily kilometers of interstate highway driving with lane kilometers of interstates in the urbanized portions of 228 metropolitan areas. In the average metropolitan area, it found that between 1983 and 1993 lane miles grew by 32 percent while driving grew by 77 percent. Between 1993 and 2003, lane miles grew by 18 percent, and driving grew by 46 percent...
The paper also calculated the elasticities of driving in relationship to lane kilometers. An elasticity of 2 would mean a 10 percent increase in lane miles would correspond with a 20 percent growth in driving; an elasticity of 1 would mean that lane miles and driving would track closely together. The paper found that elasticities were very close to 1 with standard errors of around 0.05. Even though this is contradicted by the previously cited data showing that driving grew much faster than lane miles, this is the source of Turner’s “perfect one‐to‐one relationship.”
And Randal is likely referring to this table for his figures:We investigate the effect of lane kilometers of roads on vehicle-kilometers traveled (VKT) in US cities. VKT increases proportionately to roadway lane kilometers for interstate highways and probably slightly less rapidly for other types of roads. The sources for this extra VKT are increases in driving by current residents, increases in commercial traffic, and migration. Increasing lane kilometers for one type of road diverts little traffic from other types of road. We find no evidence that the provision of public transportation affects VKT. We conclude that increased provision of roads or public transit is unlikely to relieve congestion.
The increase in VKT and lane km on the Interstate Highway (AKA: IH) is as follows:
Change in IH VKT 1983-1993 | Change in IH VKT 1993-2003 |
4,128,000 (7,627,000) | 4,056,000 (7,328,000) |
Change in IH lane km 1983-1993 | Change in IH lane km 1993-2003 |
68 (79) | 72 (129) |
If we adjust those figures respectively for the percentage of VKT and lane km that's urbanised, we get 2,955,260 (6,317,120) km for 1983, 5,238,200 km (10,670,440) for 1993, and 7,661,000 (15,157,920) km for 2003; and 330.6 (478.5) km for 1983, 434.8 (622.4) km for 1993, and 512 (743.2) km for 2003. Now we can calculate the change in VKT in urbanised areas:
Change in IH VKT 1983-1993 (Urban) |
| |||
2,282,940 (4,353,320) | 2,422,800 (4,487,480) | |||
Change in IH lane km (Urban) 1983-1993 | Change in IH lane km (Urban) 1993-2003 | |||
104.2 (143.9) | 77.2 (120.8) |
Now we can calculate the lane km growth percentage vs VKT growth percentage:
|
| |||||
53% (46%) [77% (69%)] | 34% (30%) [46% (42%)] | |||||
Change in IH lane km % [urban] 1983-1993 | Change in IH lane km % [urban] 1993-2003 | |||||
4.1% (4.7%) [31% (30%)] | 5.9% (7.8%) [18% (19%)] |
So Randal's initial statement that:
Seems to be true based on the aforementioned figures derived from Giles & Duranton's table. This seems to contradict the 1:1 relationship suggested in the abstract:In the average metropolitan area, it found that between 1983 and 1993 lane miles grew by 32 percent while driving grew by 77 percent. Between 1993 and 2003, lane miles grew by 18 percent, and driving grew by 46 percent.
So are Giles & Duranton that idiotic or dishonest? Or did Randal miss out on some aspect of their paper, some extra data that might make sense of those figures, intentionally or not?VKT increases proportionately to roadway lane kilometers for interstate highways and probably slightly less rapidly for other types of roads.
In fact, Randal did leave out the following data from Giles & Duranton:
Here is Table 2:Table 2 reports estimates of the elasticity of MSA VKT to lane kilometers from univariate OLS regressions. Each panel considers a different type of road: MSA interstates in panel A, urbanized MSA interstates in panel B, major urban roads in panel C, and nonurban MSA interstates in panel D. Columns 1 to 3 consider the 1983, 1993, and 2003 cross sections in turn. Depending on the decade, the elasticity of MSA interstate highway VKT with respect to lane kilometers is between 1.23 and 1.25. Focusing only on interstate highways in the urbanized part of MSAs yields similar results. For major urban roads and nonurban MSA interstates, we obtain slightly lower estimates between 1.00 and 1.14.
So evidently Randal used a childishly simplistic comparison between the % change in VKT & lane km to determine the elasticity between the two variables, when an Ordinary Least Squares (OLS) regression is more appropriate in this context, and gives much different results.
Now I don't know what OLS is, and the math in Giles & Duranton's paper is way above my head, but I think that two economists trained in their field probably know quite a bit more than is required to be capable of such a brazen contradiction that Randal alleges. Ergo, if you spot a contradiction - real or not - in a peer reviewed paper written by experts in the relevant field, chances are they would have done the same, as it is highly unusual for them to miss such a simple contradiction.